Calculating ROI on your Data Spend
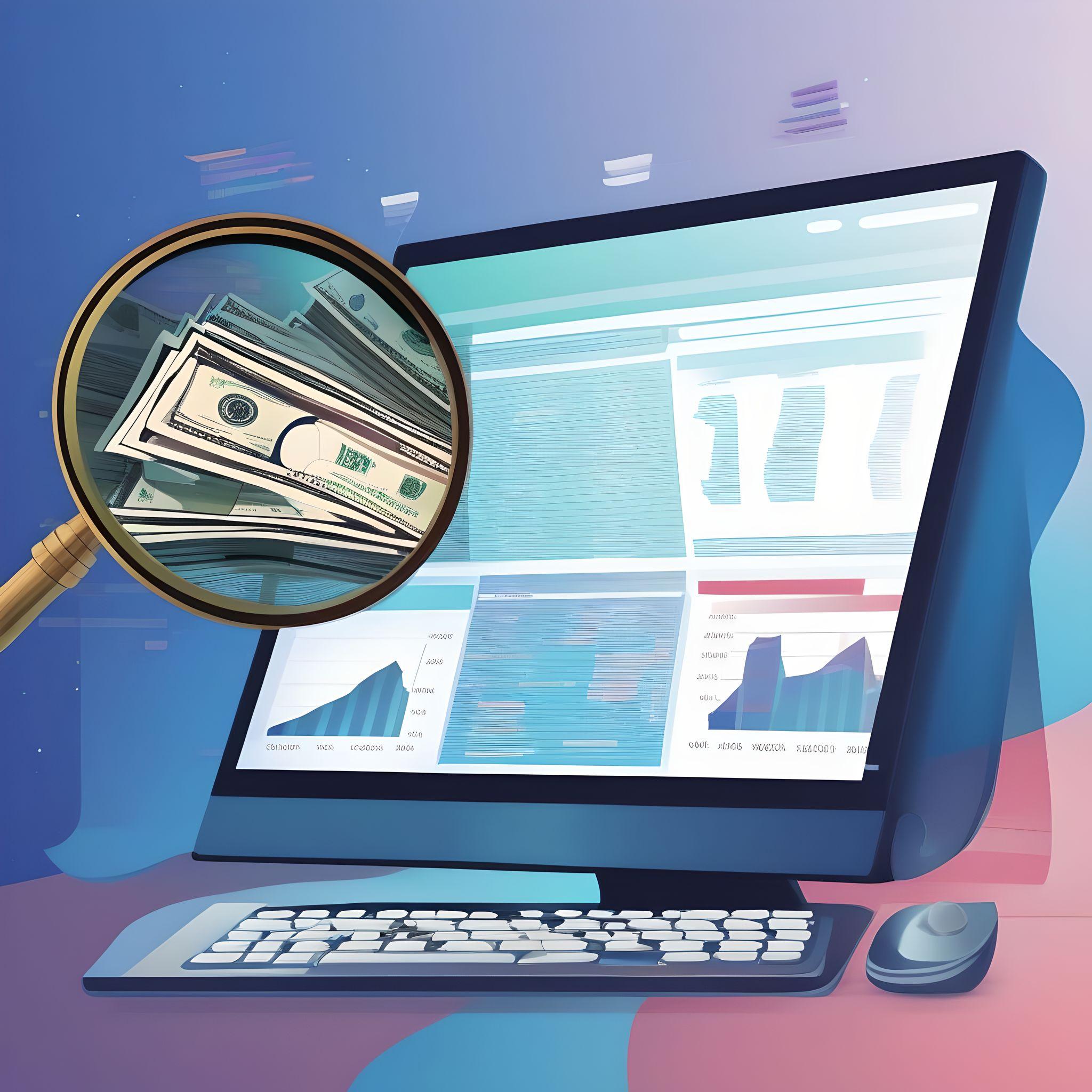
In the current "do more with less" business landscape, the reliance on data transcends mere trend—it's become a fundamental pillar supporting strategic decisions across all operational realms. From delineating new territories for market expansion to refining the precision of targeted marketing campaigns, the role of high-quality data is undeniably central. But as we navigate this data-rich environment, a crucial question emerges: How does one calculate the Return on Investment (ROI) from their engagement with data providers? Let’s explore this, not just through the lens of financial metrics but as a comprehensive evaluation of strategic value, embodying a bit of the analytical depth Ezra Klein is known for.
Calculating ROI: The Formulaic Approach
To quantify the ROI from a data provider, companies can employ several financial formulas, including the Customer Acquisition Cost (CAC) and the Magic Number. The CAC measures the cost associated with acquiring a new customer, while the Magic Number, calculated as the ratio of new revenue to the sales and marketing expenses, provides insight into the efficiency of these efforts.
A simplified formula for calculating ROI from a data provider could look like this:

Where "Revenue Attributable to Data" represents the incremental revenue generated through strategies informed by the data provider's insights.
Understanding Success in Data Initiatives
To encapsulate the ROI of data providers, it's crucial to first define what "success" means within the context of these initiatives. This definition encompasses both the direct outcomes that stem straightforwardly from a project and the indirect benefits that ripple through the organization, catalyzing further positive changes. Whether it's through enhancing sales, optimizing performance, minimizing returns, or elevating customer satisfaction and corporate reputation, the scope of success is broad and multifaceted.
Key Factors in ROI Calculation
1. Cost Reduction: Perhaps the most quantifiable benefit, cost reduction, can be directly observed through the efficiencies brought about by automation and streamlined workflows. The formula for monthly savings—calculating hours saved multiplied by the number of users and their approximate hourly cost—provides a tangible measure of the cost efficiency achieved.
2. Direct Data Monetization: The ROI from direct data monetization presents a straightforward calculation, dependent on the revenue generated from selling data. This approach shifts the focus from internal optimization to external revenue generation, highlighting the versatile value of data as an asset.
3. Increasing Revenue: Data and analytics can significantly boost revenue by informing strategic decisions, from customer segmentation for targeted marketing to predictive maintenance in the automotive sector, demonstrating the broad applicability of data insights across industries.
4. Faster Time to Market: By speeding up product development and market readiness, data initiatives not only extend the revenue-generating lifespan of a product but also position the company advantageously against competitors.
5. Freeing Up Resources: The time savings realized by automating routine tasks like data cleaning allow professionals to allocate their efforts to more complex and innovative projects, thus enhancing the organization's creative and operational capacity.
6. Security and Compliance: While these factors might be less directly tied to revenue, they represent significant cost avoidance in terms of potential losses from data breaches or regulatory fines. The investment in data security and compliance initiatives thus becomes a critical component of the broader ROI calculation.
The Strategic Value of High-Quality Data
Proper Territory and Market Size Planning: Good data serves as the cornerstone for identifying viable markets and sizing them accurately. It equips companies with the insights needed to avoid the pitfalls of over or underestimating market potential, thereby optimizing resource allocation. For instance, a data-driven approach enables a company to pinpoint a previously unnoticed demographic showing high engagement rates, leading to targeted product development and marketing strategies.
Time Savings and Error Reduction: The adage "time is money" holds especially true in sales. Data that offers up-to-date contact information, firmographic details, and customer preferences saves sales reps hours of prospecting and follow-up time. This efficiency not only reduces operational costs but also minimizes the errors associated with outdated or inaccurate data, thereby enhancing overall sales effectiveness.
Fueling Pipeline Creation and Revenue Growth: At the heart of every successful sales strategy lies a robust pipeline fueled by quality leads. High-quality data ensures that the sales team's efforts are directed toward the most promising prospects, thereby accelerating the sales cycle and boosting revenue. For example, leveraging data insights to refine lead scoring models can significantly improve conversion rates.
Marketing Efficiency and Brand Awareness: Marketing teams rely heavily on data to craft campaigns that resonate with their intended audience. Accurate data allows for the creation of detailed buyer personas, ensuring that marketing messages hit the mark and effectively contribute to achieving MQL goals. Moreover, understanding the nuances of your Ideal Customer Profile (ICP) through data can dramatically increase brand awareness and loyalty within your target segments.
Identifying Competitor Risk and Churn Prediction: In the dynamic battlefield of market competition, data on shifts within your client base or among key decision-makers can provide early warnings of potential churn or competitive encroachment. By monitoring these indicators, companies can proactively address concerns and reinforce client relationships, safeguarding their market position.

The Role of AI in Enhancing Data Quality
The transformative impact of Artificial Intelligence (AI) and machine learning on data quality and analysis marks a significant milestone in the journey towards more informed and strategic decision-making processes. As these technologies continue to evolve, they have become instrumental in sifting through the exponential amounts of data generated every day, extracting actionable insights with a level of precision and efficiency previously unattainable. AI algorithms stand at the forefront of this revolution, showcasing an unparalleled ability to detect patterns, trends, and anomalies within sprawling datasets, thereby enhancing both the reliability and relevance of the information at hand.
One of the most compelling endorsements of AI's role in improving data quality comes from Andrew Ng, a globally recognized leader in AI and co-founder of Google Brain, who famously stated, "AI is the new electricity. Just as electricity transformed almost everything 100 years ago, today I actually have a hard time thinking of an industry that I don’t think AI will transform in the next several years." This analogy not only highlights AI's transformative potential but also underscores its capacity to revolutionize how we harness, analyze, and derive value from data.
Ng's perspective is particularly relevant when considering the impact of AI on data quality. By "transforming" raw, often chaotic data into a structured, meaningful form, AI enables organizations to unlock deeper insights and more accurately predict future trends. This process is akin to filtering a murky stream into clear, drinkable water, making it infinitely more useful for a wide range of applications. Furthermore, AI-driven tools and platforms are increasingly adept at cleaning data, filling in missing values, and even identifying data that may be erroneous or misleading, thereby significantly reducing the margin for error in data-driven decisions.
The strategic value of AI-enhanced data cannot be overstated. In an era where data-driven strategies underpin competitive advantage, the ability to quickly and accurately analyze vast datasets is invaluable. AI not only augments the direct Return on Investment (ROI) from data initiatives by enabling more nuanced and sophisticated analyses but also plays a pivotal role in uncovering opportunities for innovation, cost reduction, and enhanced customer experiences. These capabilities ensure that organizations are not merely reacting to market dynamics but are anticipating changes, staying several steps ahead of the competition.
The Case for Building In-House Data Curation
While purchasing data from providers is a common practice, there's a compelling argument for developing in-house data curation capabilities. By tailoring data collection and analysis processes to their specific operational context, companies can significantly enhance the relevance and applicability of their data, thereby improving ROI. For instance, creating a bespoke data curation system that integrates real-time customer feedback can enable more agile adjustments to product offerings and marketing strategies, driving higher revenue growth. Blending first and third party data in order to compile a comprehensive and more enlightened view the market and your audience has tremendous business implications.
As renowned author and consultant Geoffrey Moore put it, "Without big data analytics, companies are blind and deaf, wandering out onto the Web like deer on a freeway."
Moore's analogy vividly highlights the critical importance of data quality, not just for its inherent value but as an indispensable tool for revenue teams navigating the complex and fast-paced digital marketplace. High-quality data equips organizations with the insights needed to make informed decisions, avoid missteps, and strategically position themselves for success.
In essence, the journey toward realizing maximum ROI from data investments is multifaceted, blending financial calculation with strategic evaluation. High-quality data, enhanced by AI and customized through in-house curation, forms the bedrock upon which successful business strategies are built. In this data-driven era, the organizations that master the art of leveraging data effectively will not only navigate the complexities of the market more adeptly but also carve out a competitive edge that is both sustainable and dynamic.